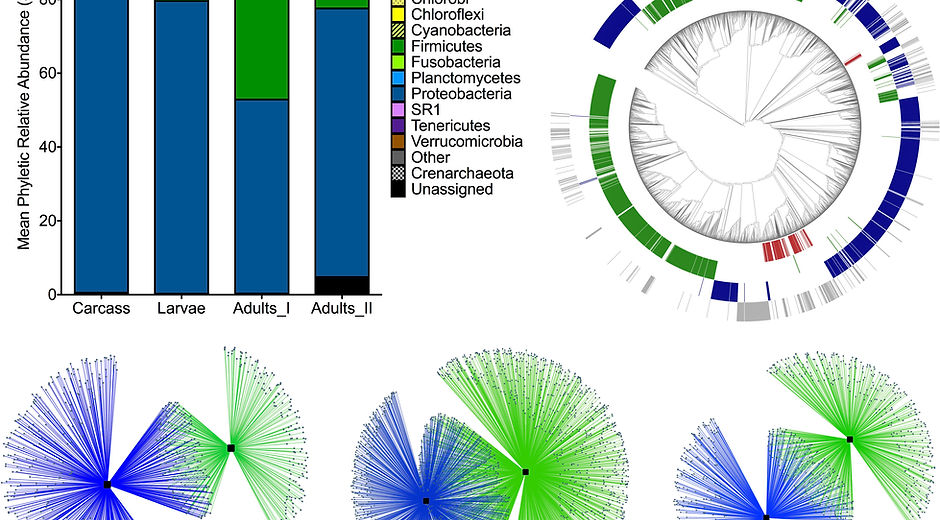
Data Analytics
The ecological datasets from decomposition studies resulting are usually large, complex, and dynamic. Thus, we employ statistical models to study the populations of microbes, insects and/or their interactions as decomposition occurs in natural habitats. Additionally, this growing toolbox of analytical and computational approaches are used in collaboration with scientists that have research interest outside of decomposition.
​
We ​focus on the power of large data and computation tools to develop models that identify ecological interactions between insects and microbiomes involved with decomposition in dynamic environments. We are developing models to identify abiotic and biotic factors that influence community assembly during decomposition, which can alter this vital ecosystem process.
The microbes identified from targeted amplicon or metagenomic high-throughput sequencing data. Bioinformatic tools are then used to characterize microbial populations during decomposition of organic material. These data can then be used, in conjunction with or independently of insect data, to assess biodiversity and function across spatial and temporal scales.
​
We often use statistical models, such as machine learning or stochastic discrimination approaches, to identify key taxa that could predict the length of decomposition time. Our models can be tested in real-world applications, such as death investigation in forensics.
The characterization of the structure, function, and interactions of microbes and insects within dynamic biological systems is vital for assessing variation in the real world. Overall, our work is prioritizing the evaluation of interdisciplinary datasets to tease apart complex ecological networks associated with decomposition, and optimize this knowledge to benefit the living.
​
​